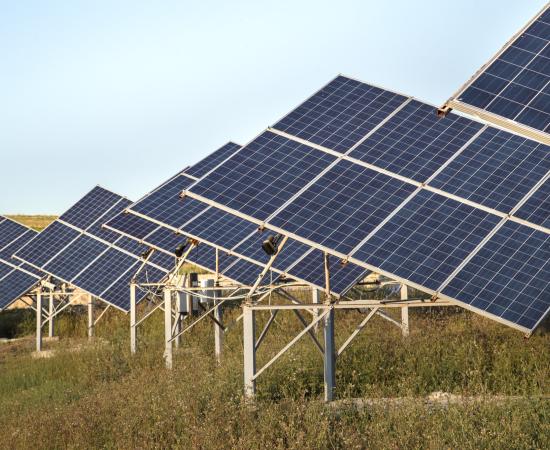
FleetAId | Fleet-based artificial intelligence for data-driven condition monitoring of industrial assets
The project aimed to create an AI-enriched research environment that supports and facilitates exploitation of fleet-based data for maximising fleet performance. This environment includes advanced data processing technologies related to integrative modelling, active learning and transfer learning which are applied for root cause analysis and continuous performance monitoring and are validated through the development of several industrial prototypes for the PV domain.
Context
Nowadays many companies operate and manage large groups of complex industrial assets which are similar in kind but different in terms of their concrete specifications. Assets can be geographically distributed but often subsets of assets are co-located. Examples are photovoltaic (PV) equipment organised within plants, wind turbines arranged within wind power plants, compressors and pumps in industrial surroundings, or fleets of utility vehicles.
Usually the enormous amount of data that is generated by such a fleet of assets is underexploited. Most often, this data is merely used by maintenance and service personnel for reactive maintenance. Some lead user companies have recently shifted to proactive maintenance, but even in these cases the analysis is realised at individual machine level. This leaves a huge potential of untapped exploitation opportunities at fleet level: traditional AI methods do not exploit important intrinsic characteristics associated to the fleet aspect, i.e. the immense richness of the data originating from the heterogeneity inherent to a fleet, and the hierarchical composition present between the various components in an asset and the various assets in a fleet.
In this context, FleetAId aimed to create an AI-enriched research environment that supports and facilitates semi-automatic exploitation of fleet-based data for maximising the performance of large and heterogeneous fleets of complex industrial assets in operation. The realised AI technologies were demonstrated through the development of several industrial prototypes for the PV domain.
Objective and results
FleetAId demonstrated the developed AI functionalities through the development of several industrial prototypes for the PV domain through three concrete challenges in commercial PV plant operations:
- optimised design choices based on context-sensitive (asset, plant, environment) performance benchmarking within fleets,
- operating cost reduction through reliable automatic detection of performance degradation and component faults, and
- reliable automatic root cause diagnosis.
Funding
- Funding agency: Innoviris
- Modality: Team Up (Artificial Intelligence call, Innoviris)
This project has been funded by the Brussels-Capital region. Innoviris is the public organisation that supports and funds research and innovation in the Brussels-Capital Region.